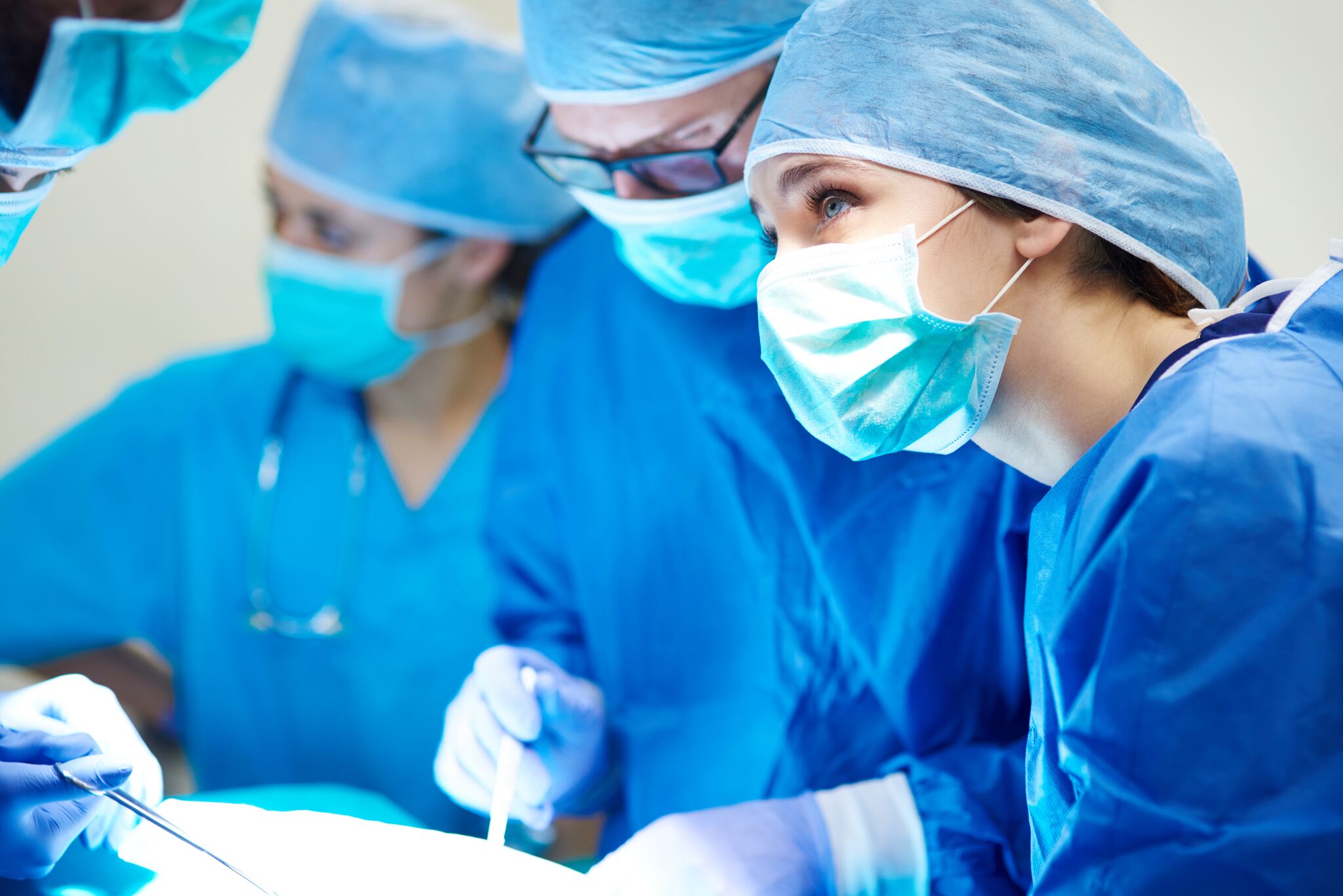
Alongside clinical outcomes, patient satisfaction is at the core of delivering effective healthcare. An area of emphasis within this realm is the perioperative care spectrum, which refers to patient treatment before, during, and after surgery. At each of these stages, opportunities are present to enrich the patient’s experience, from reducing the patient’s wait-time to improving patient-physician communication, as well as to impact clinical outcomes to the patient, as well as viable cost savings to the hospital. Thusly, operational analytics are rising in prominence as an effective tool for measuring, addressing, and impacting patient experiences throughout the perioperative care cycle.
In this reference, operational analytics includes quantitative factors such as census, site of admission, and average length of stay, as well as qualitative variables, such as the evaluation of patient satisfaction at each stage of the perioperative cycle1. At the hospital level, operational analytics can be leveraged to elucidate the trends in order to identify relationships between operational features and patient satisfaction. More specifically, operational analytics are useful for generating data on the patient’s journey throughout the perioperative care cycle, allowing for areas of intervention. Although specific operational factors are subject to variation by patient population, location, and healthcare system, there are several specific operational analytics that can be applied to most surgical settings.
Hospital census, or the number of patients at a given time, is a variable of interest for practice management2. For anesthesiologists and surgeons, census is most applicable at the site of entry for surgical service, whether that refers to the patient entering pre-operative preparation or the anesthesia suite. By knowing exactly how many patients are present at each stage of the surgical algorithm, physicians and trainees can be allocated to service during high-volume times, and otherwise triaged to other services. Moreover, measuring census also has a benefit to the administrative side of the healthcare system, as it promotes the practice manager’s ability to efficiently admit patients to hospital beds. Without analytics, patients may be admitted haphazardly and beds in the surgical wing may be under- or over-utilized. However, by employing operational analytics to measure census, beds can be strategically distributed to accommodate the most emergent cases soonest, thus contributing to intelligent care delivery at the patient population level.
Operations research has largely improved the capacity of health systems to measure and address concerns within analytics variables such as census and bed management. Recently, researchers conducted a study across Hong Kong government hospitals that employed an intervention for hospital-wide master surgery schedules, optimizing for patient flow, capacity management, and resource allocation through the broad variable of measuring bed occupancy3. The researchers developed a simulation tool that integrated real-time information from multiple hospitals in order to predict each variable at different times of the day and year. Moreover, the data analytics collected were then applied to a decision-making tool for hospital practice managers, allowing for stakeholders to observe how the master surgery schedule changes over time and with patient flow. The intervention has the potential to influence clinical outcomes as well as economic outcomes over time. Such technological interventions that prioritize high-volume data collection for integration into analytics will continue to enter the dialogue on hospital operations management.
Operational analytics play a large role in optimizing the perioperative care spectrum, by increasing the data available on patient experience, while also identifying areas of improvement for the focus of physicians and practice managers — enhancing anesthesia management as well as operations of anesthesia services.
1. Institute for Healthcare Management. “Real-Time Demand/Capacity Management to Improve Flow.” 2019. http://www.ihi.org/resources/Pages/Changes/RealTimeDemandCapacityManagement.aspx
2. The American Health Information Management Association. “AHIMA’s Long-Term Care Health Information Practice and Documentation Guidelines: Practice Guidelines for LTC Health Information and Record Systems.” 2014.
3. Yip et al. “Levelling bed occupancy: reconfiguring surgery schedules via simulation.” Int J Health Care Qual Assur. 2018;31(7):864-876. doi: 10.1108/IJHCQA-12-2017-0237.